More than a feeling: Quantifying consumer sentiment
- Among a flood of alternative data sources, consumer sentiment based on online citations stood out as adding explanatory power in the cross-section of returns.
- The consumer sentiment signals examined had little correlation with traditional style-factor exposures.
- Individual metrics and the equal-weighted combination had positive factor and quintile spread returns and positive information ratios.
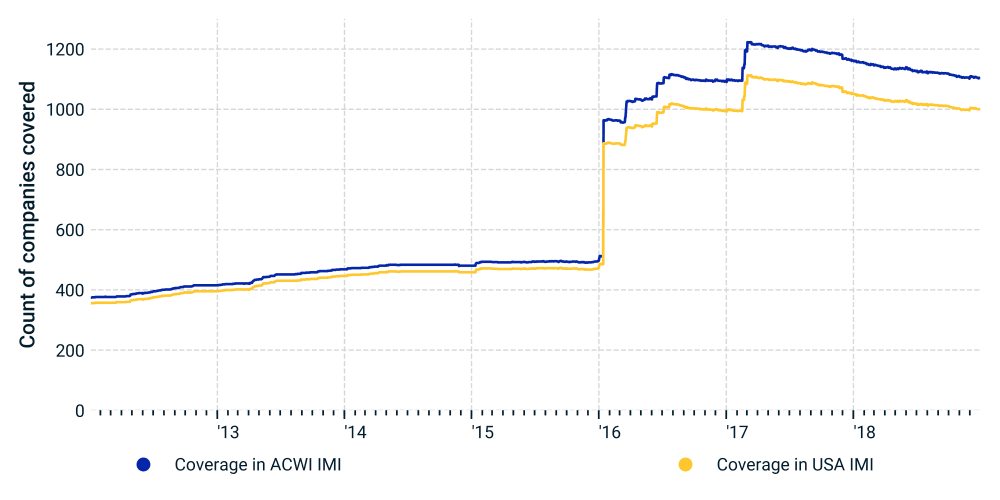
Signal | Percent Change YOY | Slope Last 91 Days | Percentile Ranking |
---|---|---|---|
Signal Percent Change YOY | Percent Change YOY 1.0 | Slope Last 91 Days None | Percentile Ranking None |
Signal Slope Last 91 Days | Percent Change YOY 0.28 | Slope Last 91 Days 1.0 | Percentile Ranking None |
Signal Percentile Ranking | Percent Change YOY 0.21 | Slope Last 91 Days 0.65 | Percentile Ranking 1.0 |
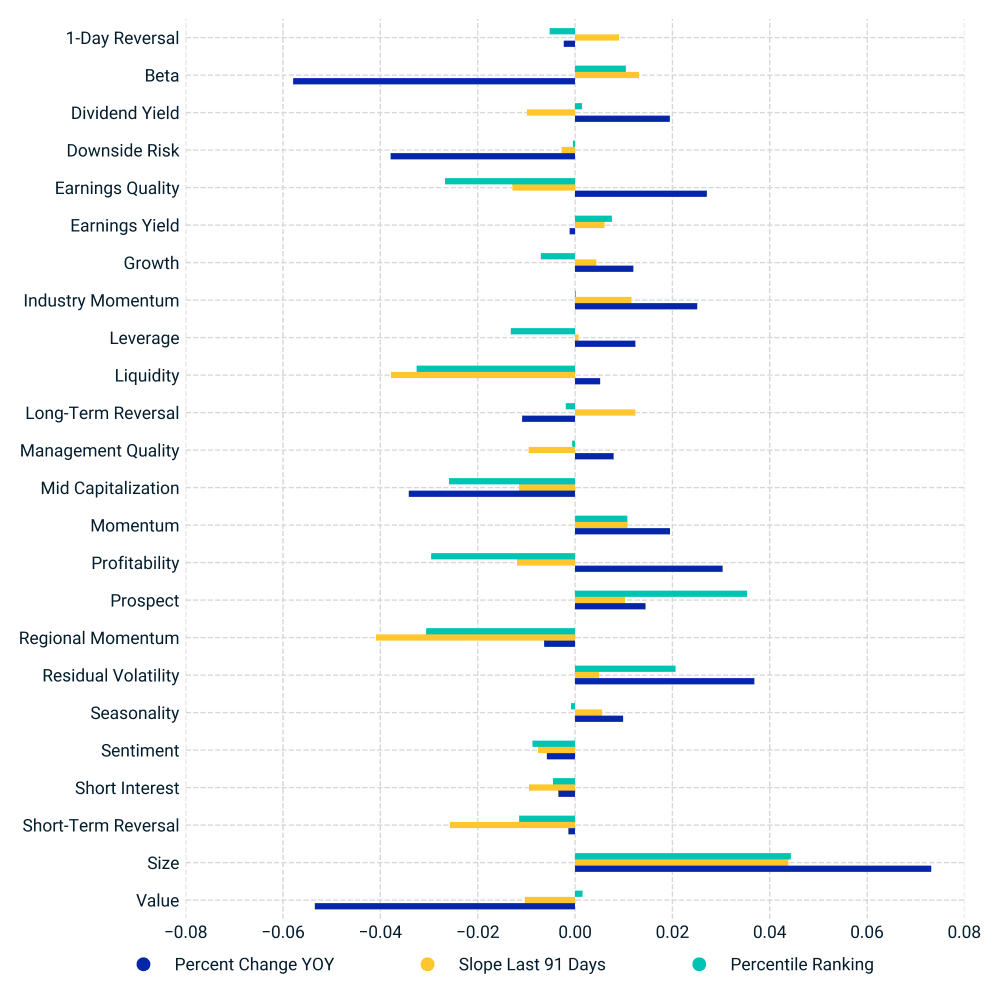
Unnamed: 0 | Quintile Portfolio Statistics | Quintile Portfolio Statistics.1 | Quintile Portfolio Statistics.2 | Multivariate Regression Statistics | Multivariate Regression Statistics.1 | Multivariate Regression Statistics.2 | Multivariate Regression Statistics.3 | Multivariate Regression Statistics.4 | Stability |
---|---|---|---|---|---|---|---|---|---|
Unnamed: 0 Signal | Quintile Portfolio Statistics Quintile Spread Return, % | Quintile Portfolio Statistics.1 Quintile Spread IR | Quintile Portfolio Statistics.2 Rank IC (%) | Multivariate Regression Statistics Factor Return, % | Multivariate Regression Statistics.1 Factor Volatility, % | Multivariate Regression Statistics.2 Factor IR | Multivariate Regression Statistics.3 Mean |t| | Multivariate Regression Statistics.4 |t| > 2, % | Stability Exposure auto-correlation |
Unnamed: 0 Percent Change YOY | Quintile Portfolio Statistics 0.85% | Quintile Portfolio Statistics.1 0.16 | Quintile Portfolio Statistics.2 1.57% | Multivariate Regression Statistics 0.75% | Multivariate Regression Statistics.1 1.17% | Multivariate Regression Statistics.2 0.64 | Multivariate Regression Statistics.3 0.98 | Multivariate Regression Statistics.4 11.26% | Stability 0.92 |
Unnamed: 0 Slope Last 91 Days | Quintile Portfolio Statistics 1.98% | Quintile Portfolio Statistics.1 0.5 | Quintile Portfolio Statistics.2 0.81% | Multivariate Regression Statistics 0.61% | Multivariate Regression Statistics.1 1.02% | Multivariate Regression Statistics.2 0.6 | Multivariate Regression Statistics.3 0.91 | Multivariate Regression Statistics.4 10.99% | Stability 0.55 |
Unnamed: 0 Percentile Ranking | Quintile Portfolio Statistics 2.49% | Quintile Portfolio Statistics.1 0.67 | Quintile Portfolio Statistics.2 0.64% | Multivariate Regression Statistics 0.36% | Multivariate Regression Statistics.1 0.99% | Multivariate Regression Statistics.2 0.36 | Multivariate Regression Statistics.3 0.91 | Multivariate Regression Statistics.4 7.97% | Stability 0.63 |
Unnamed: 0 Eq-Wt Combination | Quintile Portfolio Statistics 2.35% | Quintile Portfolio Statistics.1 0.59 | Quintile Portfolio Statistics.2 1.19% | Multivariate Regression Statistics 0.68% | Multivariate Regression Statistics.1 1.05% | Multivariate Regression Statistics.2 0.65 | Multivariate Regression Statistics.3 0.91 | Multivariate Regression Statistics.4 8.79% | Stability 0.71 |
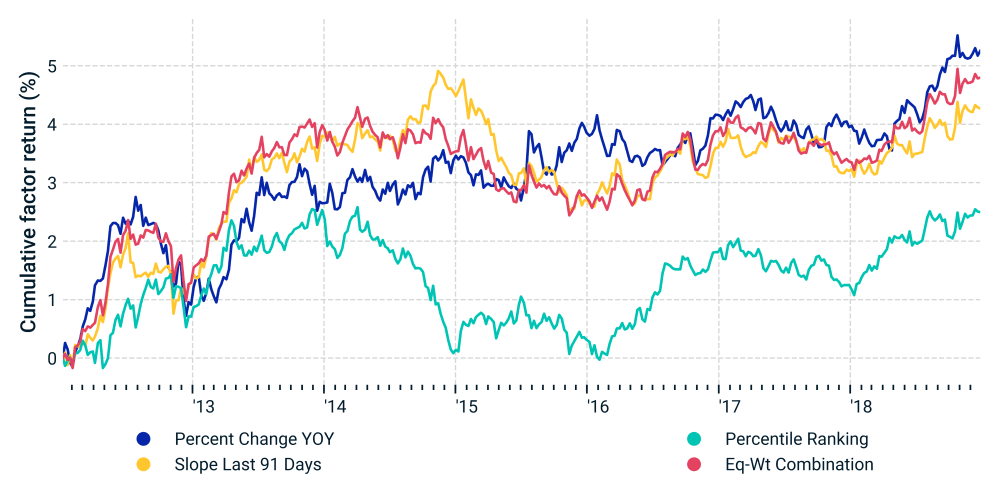
- Percent Change YOY: year-over-year percentage change (to two decimal places) in the daily number of web-based consumer brand citations, averaged over the trailing 91 calendar days.
- Slope Last 91 Days: regression slope of the year-over-year change in the number of web-based consumer brand citations over the last 91 calendar days.
- Percentile Ranking: the percentile ranking (0-100) of the citation share growth of the brand names for each company on that given day. Percentile ranking is among the entire BrandLoyalties universe, calculated via an algorithm that weights both YOY change and 91-day slope of citation share.
The content of this page is for informational purposes only and is intended for institutional professionals with the analytical resources and tools necessary to interpret any performance information. Nothing herein is intended to recommend any product, tool or service. For all references to laws, rules or regulations, please note that the information is provided “as is” and does not constitute legal advice or any binding interpretation. Any approach to comply with regulatory or policy initiatives should be discussed with your own legal counsel and/or the relevant competent authority, as needed.