MBS prepayment modeling: AI 1, Humans 0?
Blog post
September 27, 2019
- Artificial intelligence can reduce model fitting times from months to hours, significantly improving modeling efficiency and enabling true model back-testing and timelier understanding of prepayment trends.
- Our AI prepayment model has demonstrated higher model accuracy and agility than those of traditional models. It overcame high-dimensionality and high-nonlinearity issues associated with prepayment modeling..
- The AI prepayment model was able to detect new and often subtle prepayment signals that eluded traditional modeling approaches.
The limits of traditional modeling
As we discussed in an earlier journal paper, modeling prepayment rates in agency MBS is highly complex, and machine learning may lend itself to the task for several reasons.1
- The quantity of mortgage data: There are hundreds of millions of agency loans, issued across many decades.
- A large number of risk drivers at both the pool and security level: Prepayment behaviors are influenced by many macroeconomic and regional economic factors, as well as by large sets of characteristics specific to loans, borrowers and underlying properties. Dimensions of risk drivers range from 30 to more than 100. In recent decades, researchers have drawn on big data to identify alternative risk drivers for different borrowers and properties. The dimensions of these additional risk factors are often many orders of magnitude bigger than conventional model inputs.2
- Highly nonlinear and interactive risk drivers: The relationship between prepayment intensity and these numerous risk factors are often highly nonlinear and interactive. For example, when loan-note rates were above prevailing mortgage rates, borrowers would have saved money by refinancing, and prepayment intensities tended to increase with loan size, albeit to certain loan-size inflection points. However, when loan-note rates were below prevailing mortgage rates, and borrowers prepaid mortgages mainly due to house sales, prepayment tended to decrease with loan size.
- Statistical noise: Prepayment models forecast prepayment intensity, while the prepayment event itself is generally binary. Hence, fitting a model to prepayment data and assessing model accuracy are typically complex problems.
- Regime changes: Changes in financial and mortgage regulations and mortgage-business practices, along with changes in borrowers' behavior, can make consistent modeling difficult. Examples include federal loan-modification programs, such as the Home Affordable Modification Program (HAMP) and several iterations of the Home Affordable Refinance Program (HARP), as well as mortgage credit's significant tightening from 2007 to 2012 and subsequent gradual loosening.
- The idiosyncratic nature of modeling: Due to high-dimensionality and high-nonlinearity issues, prepayment models' specifications have tended to be iterative and idiosyncratic. For that reason, prepayment models often lacked transparency. Existing industry prepayment models often exhibit large and unexplained discrepancies in output, even though they are keyed off the same input data. In addition, traditional models' heavily manual process often takes months, too long for testing alternative modeling assumptions. A strict "out of sample" testing of prepayment models is generally considered unpractical.3
Complex drivers and statistical noise in mortgage prepayments
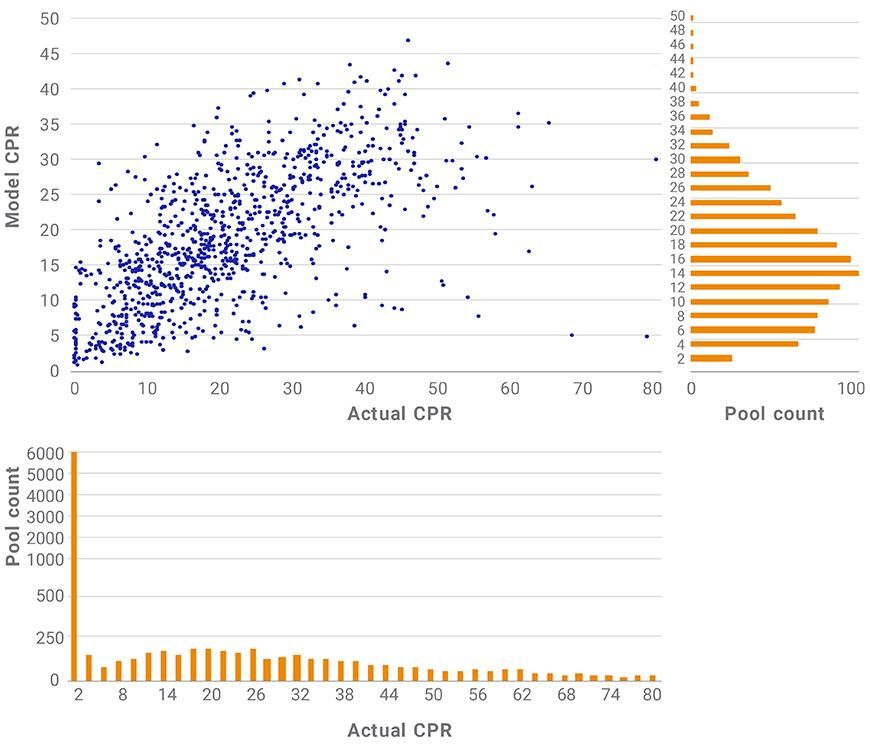
The exhibit above shows prepayment performance of a sample of 10,000 random agency 30-year pools with a 4% coupon and 90-100 basis-point incentive between 2010 and 2018. In the scattergraph, actual prepayment speeds in the conditional prepayment rate (CPR) are plotted against MSCI production-model results, as a proxy for true prepayment intensity.4 The histograms (bar charts) show the distribution of the model and actual prepayment speeds. Even with the tight band of refinance incentives, model speeds ranged from 0-52 CPR, due to variations in numerous risk drivers — e.g., loan attributes and macroeconomic and regional economic variables. The range for the actual prepayment speeds, 0-100 CPRs, was even wider, due to numerical noise. Prepayment modeling, distilling a very high-dimensional mathematical function from a large set of data amid large statistical noise, remains challenging to the modeling community after more than 20 years of effort.
AI's potential
Our pioneering AI-based model showed potential to overcome these modeling difficulties. Our AI model's results illustrated three key breakthroughs:5
- The modeling process was more efficient and standardized. Instead of the multimonth time span required for the traditional manual modeling process, the AI model fitting took hours. This efficiency gain enabled improved model backtesting, as well as a timely understanding of prepayment trends. In addition, the model results were not sensitive to the model's technical specification — and thus might have reduced modeling idiosyncrasy.
- The model displayed high accuracy and agility, which overcame the high-dimensionality and high-nonlinearity issues.
- The model detected new and often subtle prepayment signals.
Further Reading
1Zhang, D., Zhao, X., Zhang, J., Teng, F., Siyu, L., and Li, H. 2019. “Agency MBS Prepayment Model Using Neural Networks.” 2See, for example: Zhang, D. and Tang, H. “Did the U.S. Housing Crisis Start in 2003? The Impact of Borrower Subsequent Debt.” Journal of Structured Finance. The article shows that borrowers’ overall indebtedness, revealed by data from credit bureaus, is needed to understand prime mortgage defaults during the last financial crisis.3For details, see Page 2 of “Agency MBS Prepayment Model Using Neural Networks.”.4Yu, Y. 2018. "MSCI Agency Fixed Rate Refinance Model."5For details, see Pages 4-12 of “Agency MBS Prepayment Model Using Neural Networks.”
The content of this page is for informational purposes only and is intended for institutional professionals with the analytical resources and tools necessary to interpret any performance information. Nothing herein is intended to recommend any product, tool or service. For all references to laws, rules or regulations, please note that the information is provided “as is” and does not constitute legal advice or any binding interpretation. Any approach to comply with regulatory or policy initiatives should be discussed with your own legal counsel and/or the relevant competent authority, as needed.